Combatting Cyber Threats: The Role of Malware Machine Learning in IT Security
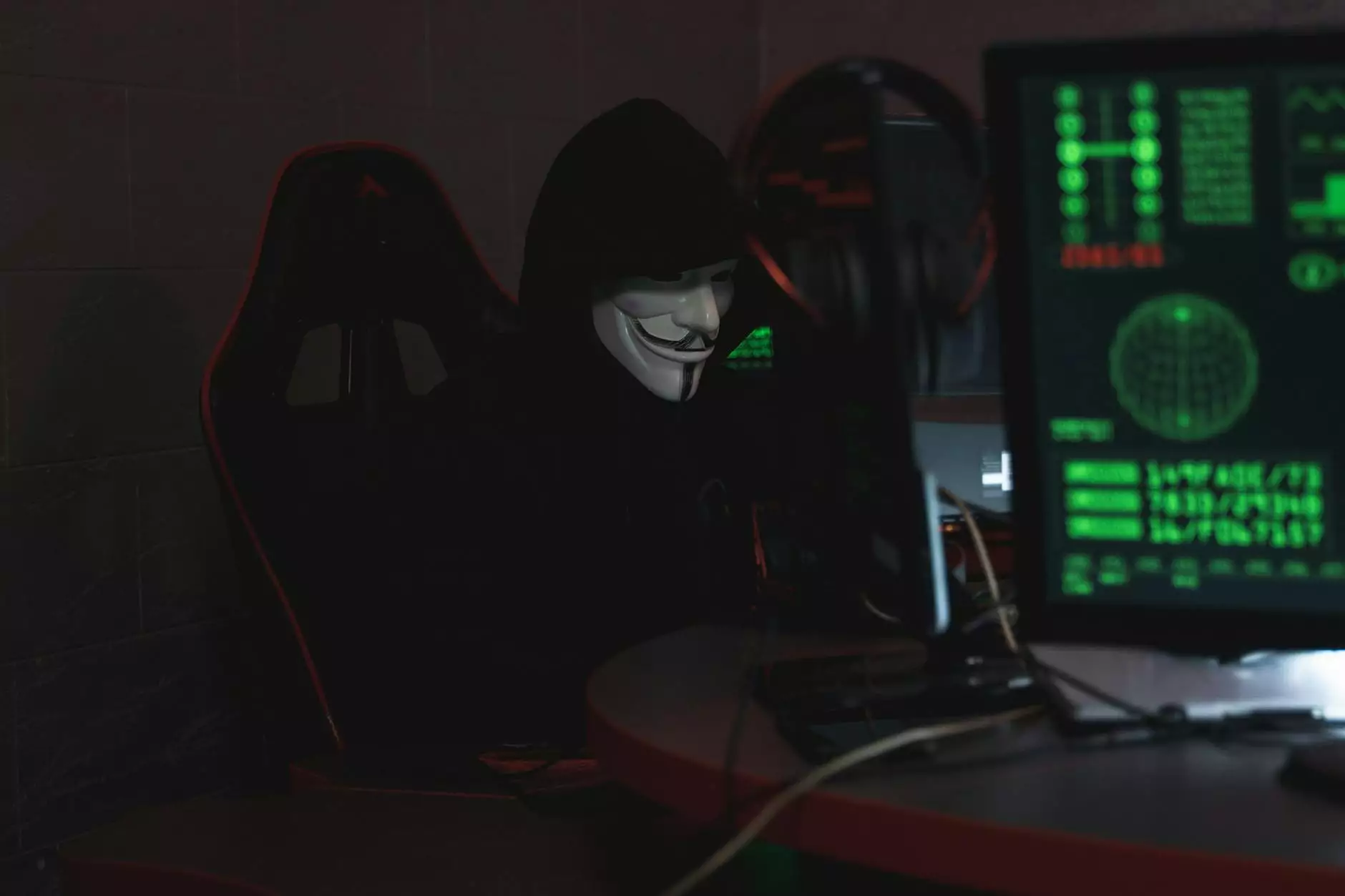
In today’s digital landscape, where businesses are increasingly reliant on technology, cyber threats are becoming more sophisticated and prevalent. In this context, understanding the concept of malware machine learning is essential for organizations that aim to strengthen their cybersecurity posture. This article delves into the intricacies of malware machine learning, its significance in IT services, and how businesses can leverage it to protect against malicious software attacks.
The Intersection of Machine Learning and Cybersecurity
Machine learning, a subset of artificial intelligence (AI), enables systems to learn from data patterns and enhance their performance without being explicitly programmed. When applied to cybersecurity, machine learning algorithms can analyze vast amounts of network traffic and user behavior data to identify anomalies indicative of potential security threats.
Given the exponential growth of malware and the complexity of cyber threats, relying solely on traditional methods for cybersecurity is no longer sufficient. This is where malware machine learning comes into play, offering a more dynamic and proactive approach to threat detection and mitigation.
Understanding Malware and Its Evolution
Malware, short for malicious software, encompasses various harmful programs such as viruses, worms, Trojan horses, and ransomware. Over the years, malware has evolved significantly, becoming more sophisticated and harder to detect. Cybercriminals continuously adapt their techniques, incorporating evasion tactics to outsmart traditional security measures.
For instance, earlier forms of malware were often straightforward and could be detected using signature-based methods. However, as malware has evolved, it has increasingly employed advanced techniques like polymorphism, where the malware changes its code to avoid detection. This evolution necessitates the adoption of advanced technologies like machine learning.
How Does Malware Machine Learning Work?
At its core, malware machine learning employs algorithms that analyze data in real-time to identify and classify potential threats. The process typically involves the following steps:
- Data Collection: Relevant data from various sources, including network traffic, file behaviors, and historical malware samples, is collected for analysis.
- Feature Extraction: Key characteristics (features) of the data are extracted. These features are essential for training machine learning models.
- Model Training: Machine learning models are trained using labeled data, where the system learns to differentiate between benign and malicious activity.
- Threat Detection: Once trained, the models can analyze incoming data to detect anomalies or identify potentially harmful software.
- Continuous Learning: The system continuously learns from new data, adapting to emerging threats and improving its detection capabilities over time.
Benefits of Implementing Malware Machine Learning in Businesses
Integrating malware machine learning into an organization's cybersecurity strategy offers several important benefits:
1. Enhanced Detection Rates
Machine learning models can analyze a higher volume of data faster than human analysts. This capability leads to improved detection rates and quicker response times, minimizing the potential damage from a malware attack.
2. Proactive Threat Hunting
Unlike traditional systems that often react to known threats, machine learning can help organizations proactively identify potential vulnerabilities and threats before they manifest into serious issues.
3. Reduced False Positives
One of the challenges in cybersecurity is managing false positives, where legitimate activities are incorrectly flagged as threats. Machine learning algorithms can improve accuracy, reducing the number of false alarms and ensuring that security teams can focus on genuine threats.
4. Adaptability to Evolving Threats
Cyber threats are constantly changing, and malware writers frequently modify their tactics to evade detection. Machine learning systems can adapt to these evolving patterns, making them highly effective at identifying new types of malware.
Real-World Applications of Malware Machine Learning
Businesses across various sectors are starting to adopt malware machine learning technologies to fortify their cybersecurity frameworks. Here are some notable applications:
1. Financial Institutions
In the financial sector, where sensitive data is at stake, machine learning can help detect fraudulent transactions or access attempts in real-time, safeguarding against substantial monetary losses.
2. Healthcare
With the rise of digital health records, cybersecurity in healthcare has become paramount. Machine learning technologies are utilized to protect patient data from breaches and ransomware attacks.
3. E-commerce
E-commerce platforms are frequent targets for cybercriminals. By implementing machine learning models, these businesses can defend against payment fraud and ensure secure customer transactions.
4. Government Agencies
Government institutions employ machine learning to protect sensitive information and infrastructure from cyber threats, thereby ensuring national security.
Challenges in Implementing Malware Machine Learning
While the advantages of malware machine learning are clear, there are challenges to consider:
1. Data Privacy Concerns
Organizations must ensure that they comply with data protection regulations when using machine learning, particularly when analyzing personal data.
2. Skill Gaps
Implementing machine learning technologies requires skilled professionals who understand both cybersecurity and data science. The talent shortage in these fields can pose a barrier to successful implementation.
3. Integration with Existing Systems
Integrating machine learning systems with legacy cybersecurity solutions can be complex, requiring considerable investment in terms of time and resources.
Future Trends in Malware Machine Learning
The field of malware machine learning is rapidly evolving, driven by advancements in technology and an increasing focus on cybersecurity. Here are some future trends expected to shape the landscape:
1. Automated Incident Response
Future systems will focus on automating not only detection but also incident response, minimizing the impact of security breaches and allowing organizations to handle threats more effectively.
2. Federated Learning
Federated learning is an emerging trend where multiple organizations can collaborate by sharing insights derived from machine learning models without prematurely exposing sensitive data.
3. Explainable AI
As reliance on AI increases, there is also a growing need for transparency in decision-making processes. Explainable AI ensures that organizations understand how models reach specific conclusions, fostering trust in machine learning systems.
Partnership with IT Services for Enhanced Security
To capitalize on the advantages of malware machine learning, businesses should consider partnering with IT services that specialize in cybersecurity. Organizations like Spambrella provide tailored IT services and security solutions to help businesses defend against evolving cyber threats.
Conclusion
The integration of malware machine learning into cybersecurity measures signifies a paradigm shift in how organizations approach cyber threats. As malware continues to evolve, businesses must adopt advanced technologies to stay ahead of potential attacks. By leveraging machine learning, businesses can enhance their detection capabilities, reduce false positives, and ultimately protect their vital assets and reputation in an increasingly digital world.
Investing in comprehensive IT services and robust security systems ensure not only the safeguarding of business data but also the trust and confidence of customers in the digital age. Embrace the future of cybersecurity with innovation and vigilance as your guides.